As the economy takes a downturn in the second quarter of 2022, and gloomy projections of the market have translated into investors becoming more conservative in their movements, early stage seed startups in healthcare have been slowly beginning to feel the effects. Whereas the digital health, biotech, and life sciences industries have long required more due diligence in respect to their technology and more scrutiny in consideration of the consequences of their failures to their clients, investors in healthcare, much like their counterparts in other fields have reduced their risk appetite even further. In the first quarter, VC funding in life sciences slowed to $6.5 billion, a two year low according to The Business Journal.
Where investment activity has largely been sustained, as of yet, is in software based digital health: Saas products have considerably lower burn and lower product life cycle. A large fraction of those companies rely on AI as a driving force and selling point to their customers.
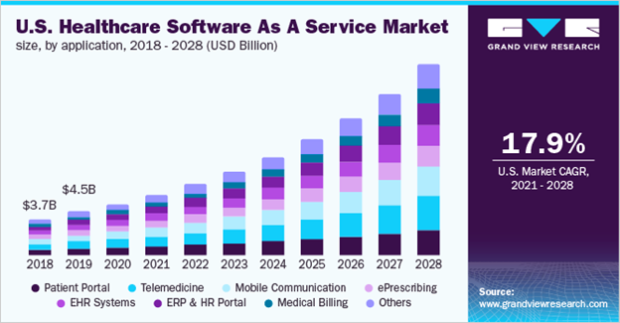
However, AI is increasingly becoming a commodity: no longer is the question of who can do AI, and what the iteration speed is, the ways to leverage it become increasingly more important. This brings us to a couple points:
- Companies such as Scale AI are democratizing access to cutting edge algorithms
- This allows focus on non technicals hires as a better investment,
- This provides startups with a choice between hiring third party (which may be cheaper), or hiring technical engineers
- IP (in-house) or speed (third party) becomes a relevant differentiator strategy choice to consider
Recently, Mckinsey published a report on work after COVID-19, which highlighted that “two-thirds of the senior executives surveyed are stepping up investment in AI” (in which we at Tau firmly have believed since starting our fund two years ago), the landscape of AI in healthcare has beginning to shift: value add is being brought not just through cutting edge AI algorithms and resulting insights but through generating a new generation of information and book-keeping of data such that it is usable and accessible for a variety of applications, including training algorithms. Startups can utilize these trends to do the following:
- Focus on data acquisition pipeline and partnerships
- Strategize hires not only for algorithm design but data maintenance and structural support
- Devote energy to understanding the leverage of AI within context, not just the tech itself
Note from Amit Garg: Primary author for this article is Sharon Huang. Originally published on “Data Driven Investor,” am happy to syndicate on other platforms. I am the with 20 years in Silicon Valley across corporates, own startup, and VC funds. These are purposely short articles focused on practical insights (I call it gl;dr — good length; did read). Many of my writings are at https://www.linkedin.com/in/amgarg/detail/recent-activity/posts and I would be stoked if they get people interested enough in a topic to explore in further depth. If this article had useful insights for you comment away and/or give a like on the article and on the Tau Ventures’ LinkedIn page, with due thanks for supporting our work. All opinions expressed here are my own.